Clustering: a real project to explore data
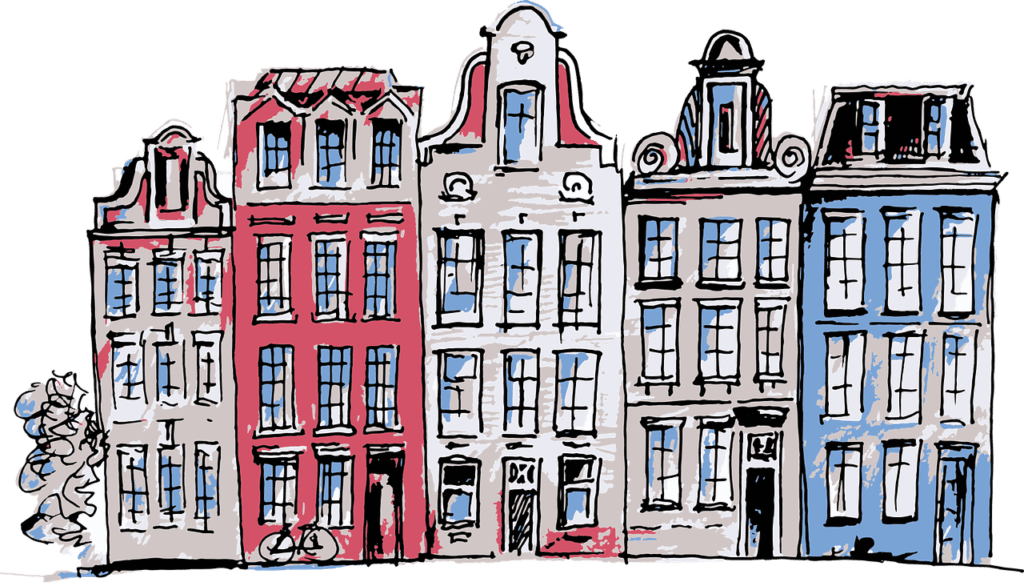
Clustering is a very powerful tool for grouping data. There are many algorithms that can be applied, so the choice is always difficult. In addition, all clustering algorithms require parameters to work. By means of a real case study, applied to real estate data, we will combine PCA, hierarchical clustering and K-means to provide optimal clustering solutions.
Hierarchical clustering: how it works
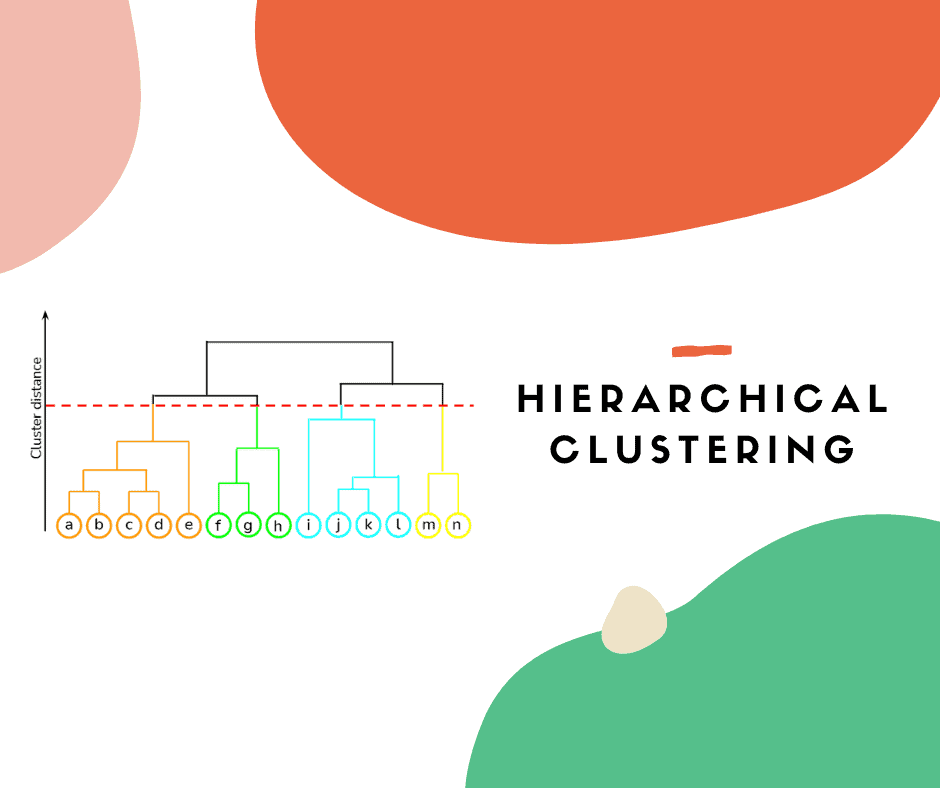
Clustering algorithms allow data to be grouped according to their inherent characteristics. There are many algorithms that have been developed over the years. Hierarchical clustering, thanks to a graphical representation called a dendogram, makes it possible to visualize at a glance the composition of clusters and interpret their characteristics. Let us find out, step by step, how it works and how to interpret the results obtained.
K-Means: how it works
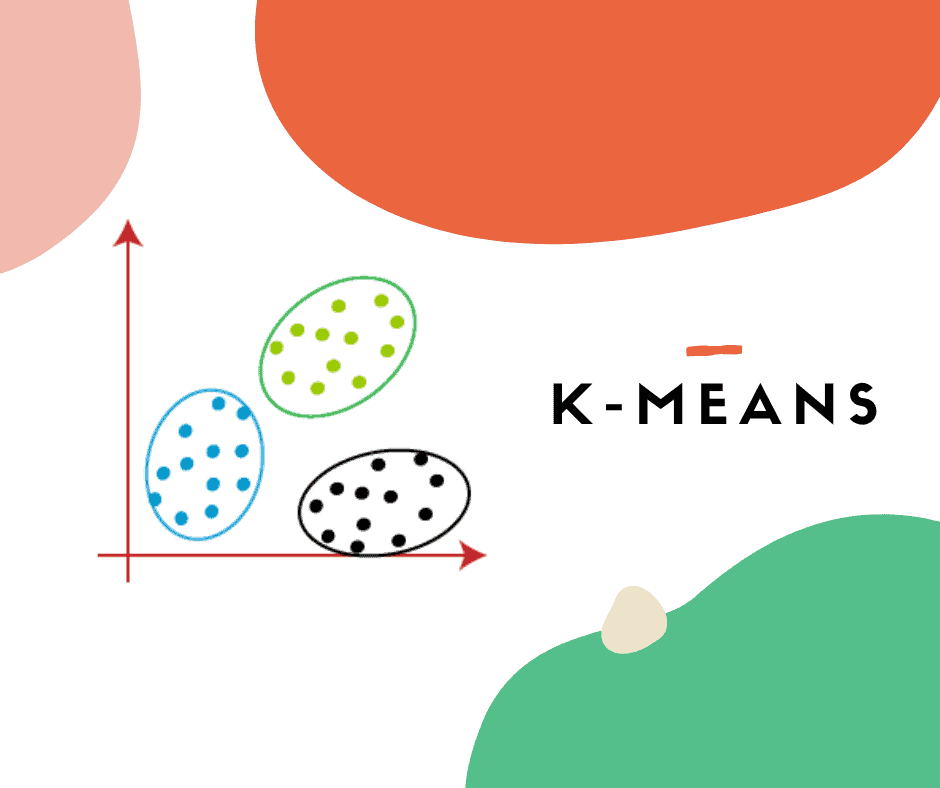
Clustering algorithms allow data to be grouped according to their inherent characteristics. There are many algorithms that have been developed over the years. K-Means is certainly the most popular and simple one. Let’s find out, step by step, how this method succeeds in identifying clusters of data using only the number of groups that need to be extracted.
DBSCAN: how it works
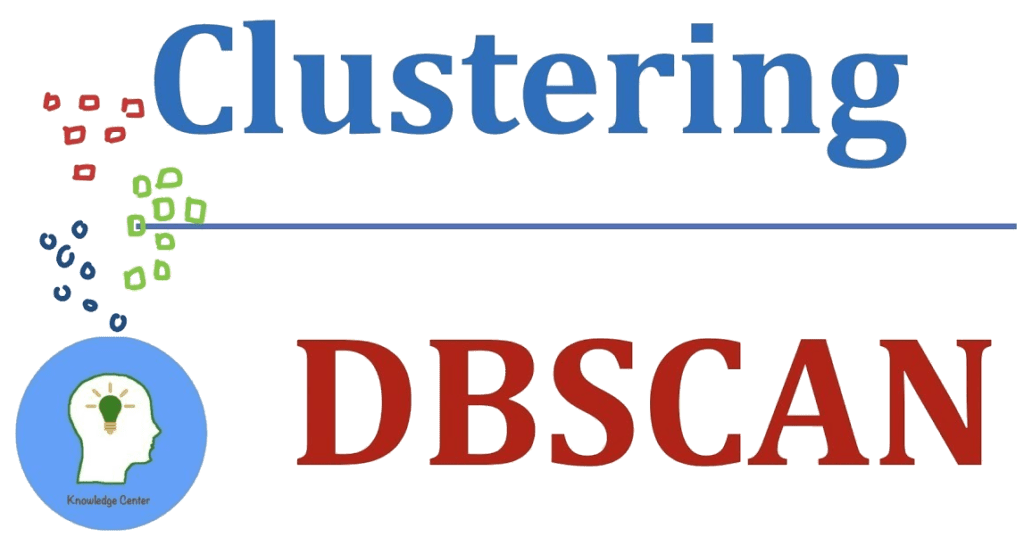
Clustering algorithms allow data to be grouped according to their inherent characteristics. There are many algorithms that have been developed over the years. Among the most famous ones, we cannot forget DBSCAN. Let’s find out, step by step, how this method succeeds in identifying clusters of data of any shape and size thanks to only two parameters.